Investing in AI: Fundamentals vs. Hype
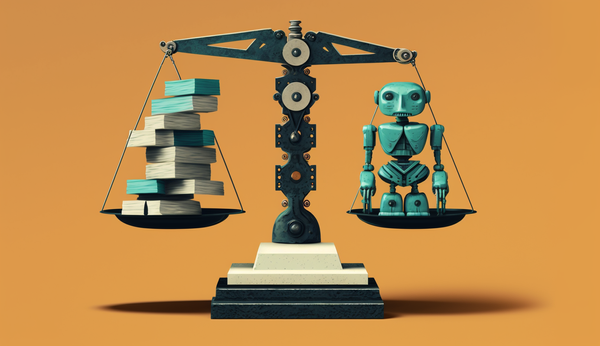
Welcome to the AI hype cycle brought to you by OpenAI, Google, Meta and LLMs. There are so many narratives going on around AI, it is hard to keep up. But in true Faculty style, we want to talk about investing in AI, current valuations, and why this reduces its VC appeal. The valuations of companies both directly building tools and the platforms for AI are being hyped, whether it is Google, Meta, Microsoft, or indirectly linked hardware builders like Nvidia, which is used to train these LLMs.
Many young AI companies are raising crazy amounts of money on insane valuations, pre-product, nevermind pre-revenue. Their whole argument: we need this level of funding because H1s and training hardware are cost-intensive. Therefore we are going to be spending somewhere between $40-$60m training our own LLM. For example, France’s MistralAI recently raised $105m on a $200m valuation a month after it was founded.
AI start-ups are coming to venture funds because the start-up costs are so high, but to avoid super dilution right off the bat to founders, their valuation has to be suitably high. This is similar to combining the first four traditional funding rounds - Pre-Seed, Seed, Series A, B & almost C to get to this level of valuation. Why would any venture capital fund front-run funding for a pre-product, nevermind pre-revenue company when the fundamentals are not there yet? This is a question for anyone investing in AI start-ups.
More importantly, what is this level of investment for? To buy hardware for them to train LLMs. These start-ups don’t have a moat, so VCs are essentially subsidising CAPEX. Are the VCs participating in these insane valuations to buy equity in a highly innovative company, or are they basically doing a leasing agreement for hardware that the start-up is going to use? A bank, not a VC, should do a leasing agreement. Fundamentally, none of it makes any sense.
AI could take a few lessons from Web3 start-ups that raised huge valuations in the last two bull cycles (2017 & 2021). Many of these start-ups raised VC money on the promise of creating a new ‘metaverse’ or ecosystem which copied traditional Web2 markets but made them decentralised. Of these companies, many wasted valuable runway on expensive hires and building technology stacks without a clear product or customer in mind. As a result, many ‘zombie’ companies were created that have not delivered revenue or user traction nor the return on investment that VCs expected.
For the last 18 months, most VCs have been sitting on the sidelines because there hasn’t been much activity in the market, as this is still very much a sideways cycle. Perhaps the motivation for these deals is that most VCs have to justify the 2% they charge in fees, and an AI mega deal is a way to show they are doing something. However, the structures of these deals are not helping anyone.
If the pursuit of Unicorns has taught us anything, it should be that the world’s most successful companies were founded in times of scarce capital allocation and based on lean start-up funding models. Here are some funding facts to keep in mind:
- Google has raised total funding of $31.4M over eight rounds. Its first funding round was for $100k in 1998.
- Facebook has raised total funding of $2.26B over 18 rounds. Its first funding round was for $500k in 2004 based on a valuation of $5m.
- Amazon has raised a total of $8.1B in funding over three rounds. Its first funding came from an initial investment of $250k from Jeff Bezos’ parents.
All of these followed the traditional structure of multiple funding rounds as their business models grew and evolved. None of these tried to raise future CAPEX on the first round based on an insane valuation.
Microsoft and Open AI spent over $400m building CHAT-GPT, but according to Moore’s law, the cost of training LLMs will come down exponentially over time. We have already seen the leaking of Meta’s LLM and the open-source training that people are doing using this smaller (by parameter size) LLM and a beefy laptop. The race to iterate and train smaller models will reduce costs even more in the next few years, and it will democratise AI as users iterate using trained models and proprietary data to produce better outcomes. This is a given.
VCs often bet on hype cycles and start-ups. However, investing in AI looks like a terrible bet to make. There is No Moat for AI (according to Google’s own Memo), no real competitive advantage, and it may be cheaper to achieve the same results in a few years. From a purely math-based view, the defensibility and probability of success for start-ups in a copy & compete environment is very low. 9 out of 10 start-ups fail; ultimately, investors need to focus on AI’s fundamentals rather than the hype.